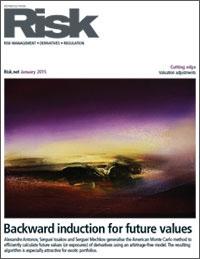
Backward Induction for Future Values
In this research paper, Drs. Alexandre Antonov, Serguei Issakov and Serguei Mechkov generalize the American Monte Carlo method to efficiently calculate future values (or exposures) of derivatives using an arbitrage-free model.
Specifically, they present efficient calculations of the portfolio values (exposure) in a self-consistent way using an arbitrage-free model that is calibrated to both implied market and real-world projections. They propose a new algorithmic method of simulation of exposures (distributions of future values) based on an iterative backward induction, a generalization of backward induction, especially attractive for exotic portfolios.
Backward Induction for Future Values
The paper applies this generalization to a simulation of exposures (distributions of future values) in the contexts of:
- Various valuation adjustments (XVAs) due to counterparty risk, funding, capital, etc.,
- Calculation of risk measures that use averages of future values, such as VAR and expected shortfall for market risk, and PFE EPE/ENE, etc. for counterparty risk.
- Scenario generation, also in real-world measure.
Overall, highlights of this article include the generalization of the American / Least Square Monte Carlo method to compute the full future value – which we call Observation Value – by backward induction. The Observation Value accounts for all scenarios, including those on which exercises do occur, i.e. scenarios on which the instrument changes.
Complete the form to download this complimentary whitepaper, “Backward Induction for Future Values”
Authors: Dr. Alexandre Antonov,Dr. Serguei Issakov, and Dr. Serguei Mechkov
In this research paper, Drs. Alexandre Antonov, Serguei Issakov and Serguei Mechkov generalize the American Monte Carlo method to efficiently calculate future values (or exposures) of derivatives using an arbitrage-free model.
Specifically, they present efficient calculations of the portfolio values (exposure) in a self-consistent way using an arbitrage-free model that is calibrated to both implied market and real-world projections. They propose a new algorithmic method of simulation of exposures (distributions of future values) based on an iterative backward induction, a generalization of backward induction, especially attractive for exotic portfolios.
Backward Induction for Future Values
The paper applies this generalization to a simulation of exposures (distributions of future values) in the contexts of:
- Various valuation adjustments (XVAs) due to counterparty risk, funding, capital, etc.,
- Calculation of risk measures that use averages of future values, such as VAR and expected shortfall for market risk, and PFE EPE/ENE, etc. for counterparty risk.
- Scenario generation, also in real-world measure.
Overall, highlights of this article include the generalization of the American / Least Square Monte Carlo method to compute the full future value – which we call Observation Value – by backward induction. The Observation Value accounts for all scenarios, including those on which exercises do occur, i.e. scenarios on which the instrument changes.
Complete the form to download this complimentary whitepaper, “Backward Induction for Future Values”
Authors: Dr. Alexandre Antonov,Dr. Serguei Issakov, and Dr. Serguei Mechkov
Authors
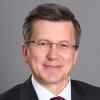
Serguei Issakov, Ph.D.
Dr. Issakov, as Chief Quantitative Officer and Senior Vice President of Global Quantitative Research and Development, oversees the company’s quantitative research globally, including the research of pricing models at Numerix. Since joining Numerix in 1999, his earlier roles at Numerix included Vice President of Financial Applications, Head of Engine Development (the forerunner to Numerix 7) and Head of Risk Analytics.
Prior to joining Numerix, Dr. Issakov held research positions in theoretical physics at the Nordic Institute for Theoretical Physics in Copenhagen, the University of Paris (Laboratory of Theoretical Physics and Statistical Models), the University of Oslo and the Center for Advanced Study in Oslo. Before that, he led research on models of brain rhythms at the Medical Radiological Center in Obninsk Russia.
Dr. Issakov has published over 40 papers in mathematics and theoretical physics. He is a co-author of the Issakov-Ouvry-Wu equations in fundamental quantum statistical mechanics. He has received numerous fellowships and research grants, including a NATO Visiting Professorship and grants from the Russian Foundation for Basic Research. He holds PhD in Theoretical and Mathematical Physics from Moscow Institute of Physics and Technology, from the Theory Group led by Physics Nobel Laureate Vitaly Ginzburg.
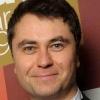
Dr. Alexandre Antonov
Dr. Antonov received his PhD degree from the Landau Institute for Theoretical Physics in 1997 and joined Numerix in 1998, where he currently works as a Senior Vice President of Quantitative Research. His activity is concentrated on modeling and numerical methods for interest rates, cross currency, hybrid, credit and CVA. Dr. Antonov is a published author for multiple publications in mathematical finance, including RISK magazine and a frequent speaker at financial conferences.