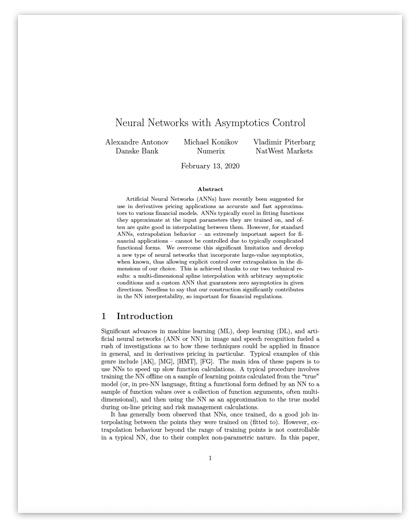
Neural Networks with Asymptotics Control
Artificial Neural Networks (ANNs) have recently been suggested for use in derivatives pricing applications as accurate and fast approximators to various financial models. ANNs typically excel in fitting functions they approximate at the input parameters they are trained on, and often are quite good in interpolating between them. However, for standard ANNs, extrapolation behavior – an extremely important aspect for financial applications – cannot be controlled due to typically complicated functional forms. We overcome this significant limitation and develop a new type of neural networks that incorporate large-value asymptotics, when known, thus allowing explicit control over extrapolation in the dimensions of our choice. This is achieved thanks to our two technical results: a multi-dimensional spline interpolation with arbitrary asymptotic conditions and a custom ANN that guarantees zero asymptotics in given directions. Needless to say that our construction significantly contributes in the NN interpretability, so important for financial regulations.
Complete the form to download this research paper, “Neural Networks with Asymptotics Control”
Authors: Dr. Michael Konikov, Dr. Alexandre Antonov, Ph.D., Dr. Vladimir Piterbarg, MD
Artificial Neural Networks (ANNs) have recently been suggested for use in derivatives pricing applications as accurate and fast approximators to various financial models. ANNs typically excel in fitting functions they approximate at the input parameters they are trained on, and often are quite good in interpolating between them. However, for standard ANNs, extrapolation behavior – an extremely important aspect for financial applications – cannot be controlled due to typically complicated functional forms. We overcome this significant limitation and develop a new type of neural networks that incorporate large-value asymptotics, when known, thus allowing explicit control over extrapolation in the dimensions of our choice. This is achieved thanks to our two technical results: a multi-dimensional spline interpolation with arbitrary asymptotic conditions and a custom ANN that guarantees zero asymptotics in given directions. Needless to say that our construction significantly contributes in the NN interpretability, so important for financial regulations.
Complete the form to download this research paper, “Neural Networks with Asymptotics Control”
Authors: Dr. Michael Konikov, Dr. Alexandre Antonov, Ph.D., Dr. Vladimir Piterbarg, MD
Authors
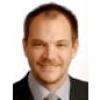
Dr. Vladimir Piterbarg
Dr. Piterbarg is a Managing Director and the Head of Quantitative Analytics at Barclays Capital. Before joining Barclays Capital in March 2005, he was a co-head of quantitative research for Bank of America, where he had worked for 8 years. Vladimir Piterbarg’s main areas of expertise are the modelling of exotic interest rate and hybrid derivatives.
Among his many published papers, Dr. Piterbarg authored "Stochastic volatility model with time-dependent skew," (Applied Mathematical Finance, 12(2): 147-185, June 2005), which introduced stochastic volatility to the LIBOR market model. He was named Quant of the Year 2006 by Risk Magazine, was the Associate Editor of the Journal of Computational Finance, and was Co-Editor (along with Leif B.G. Andersen) of the Interest Rate Modeling section for the Encyclopedia of Quantitative Finance.
Dr. Piterbarg holds a Ph.D. in Mathematics (Stochastic Calculus) from University of Southern California.
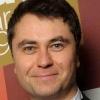
Dr. Alexandre Antonov
Dr. Antonov received his PhD degree from the Landau Institute for Theoretical Physics in 1997 and joined Numerix in 1998, where he currently works as a Senior Vice President of Quantitative Research. His activity is concentrated on modeling and numerical methods for interest rates, cross currency, hybrid, credit and CVA. Dr. Antonov is a published author for multiple publications in mathematical finance, including RISK magazine and a frequent speaker at financial conferences.
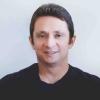
Dr. Michael Konikov
Dr. Michael Konikov is a Senior Vice President and Head of Quantitative Development at Numerix, where he manages a team responsible for the development and delivery of models in Numerix software. Previously, he worked at Citigroup, Barclays, and Bloomberg in quantitative research and desk quant roles. He completed his PhD in mathematical finance at the University of Maryland College Park, concentrating in particular on the application of pure jump processes to option pricing. Dr. Konikov's publications cover diverse asset classes ranging from equity to interest rates and credit.